DATA CARTOGRAPHY BASED AUGMENTATION TECHNIQUES FOR STANCE DETECTION
DOI:
https://doi.org/10.62229/aubinf63/93-113Keywords:
stance detection, data cartography, training dynamics, data augmentationAbstract
Stance detection is the task of determining whether the information conveyed in a text is against, neutral, or in favor of a particular target. Since there is a plethora of targets upon which one can adopt a position, one common challenge of the stance detection task is the scarcity of annotations. Conversely, the emphasis on data quantity frequently entails a compromise in terms of the quality of the data. To address both challenges, we propose two data augmentation techniques that leverage training dynamics – the model behavior on individual instances during training – to identify and combine data instances with properties that differ, triggering, for example, the improvement of the generalization capabilities of the model or the enhancement of its optimization process. The first data augmentation method uses training dynamics to generate additional virtual samples during model training by interpolating existing annotated samples with characteristics that differ. The second data annotation approach is defined as a conditional masked language modeling task that generates additional samples by predicting the masked words of the input sentence, conditioned not only on its context but also on an auxiliary sentence sampled based on its characteristics. We empirically validated that fine-tuning a pre-trained language model on a subset of the training data, such that the instances that harm the training process are excluded, achieves better performance as compared to the same model fine-tuned on the entire training dataset. Moreover, in most cases, the performance of the existing augmentation approaches was also improved by using data with properties that differ during the annotation process, as opposed to random sampling.
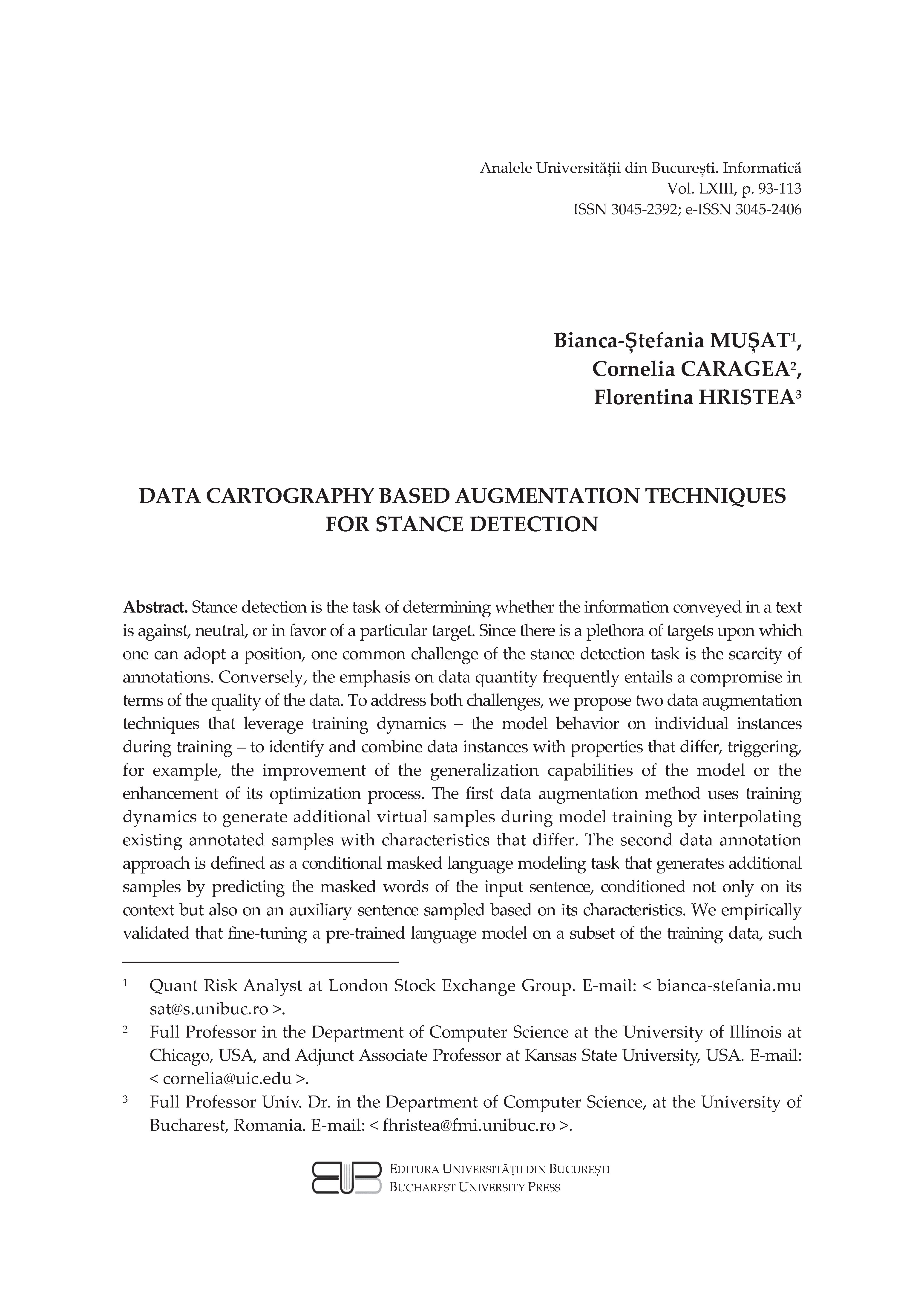