Hybrid Approach for Human Diseases Prediction Using Air Quality Index
DOI:
https://doi.org/10.25083/rbl/27.1/3270-3281Keywords:
Data Mining, CLustering In QUEst, Machine Learning, eXtremeGradiant Boosting, Air Quality Index, high performance, high-speed, accuracyAbstract
Air pollution has become an extremely serious issue as the air pollutants emitted from motor vehicles has a greater impact on human health than other contaminants. Air quality forecasting plays a major role in giving warning to people and controlling air pollution. The single technique forecasting has various drawbacks such as low accuracy, low performance and low speed. Our present work overcome the above drawbacks by using a hybrid model approach. Our proposed method aims to forecast air quality to predict the hourly concentration of air pollutants using a hybrid model of data mining and machine learning. It predicts diseases due to emission of air pollutants from the motor vehicles based on Air Quality Index level. The CLusteringInQUEst algorithm is used to cluster geo- spatial data for specific input region. The Air Quality Index (AQI) for desirable set of important air pollutant features was calculated from the datasets produced by air pollutants from atmosphere. The calculated AQI was the input to the eXtreme Gradient Boosting (XGB) decision tree. It then classifies AQI level for the specific air pollutants. Then the diseases were classified using XGB algorithm.CLIQUE method has chosen than any other data mining techniques for which it can accurately predict diseases based on AQI values. XGBoost classifier is known for its good performance gradient bosting tree models which is very fast and an efficient one for both computation time and memory. Hence the above two techniques were combined as a hybrid approach to get the benefits of those features.The hybrid model produces a result with a higher performance, accuracy and speed compared to other models. In this paper, we have compared accuracy and precision rates for the hybrid approach with two single techniques such as Support Vector Machine and Random Forest.An accuracy and Precision rates of our proposed hybrid approach was 98.6% and 98.7% than Support Vector Machine has 93.85% and 94.8% & Random Forest has 94.28% and 94.52% which proves that hybrid approach is an efficient diseases prediction technique in real-time environment.
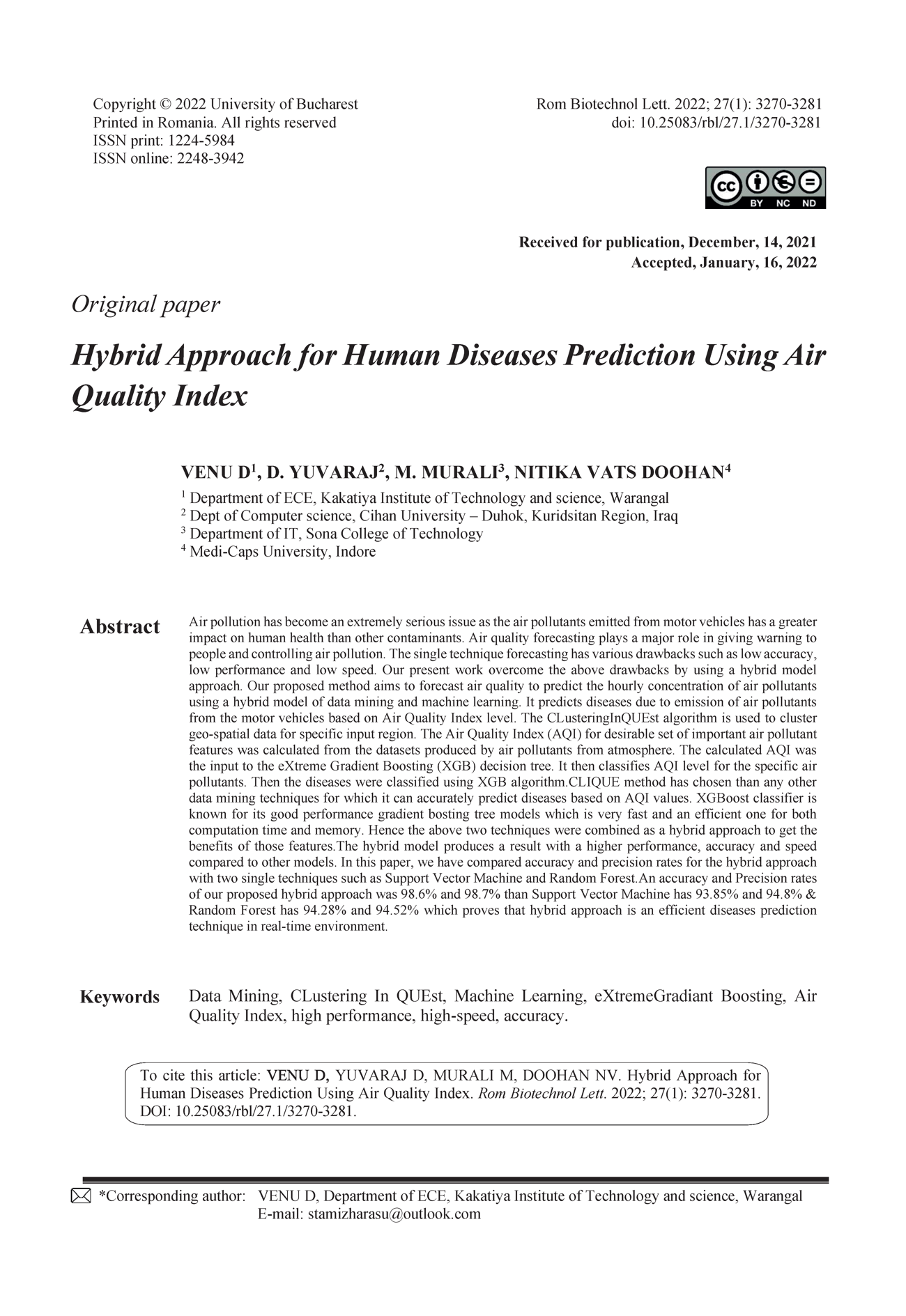