A statistical method for source approtionment of soil pollution
DOI:
https://doi.org/10.25083/rbl/28.3/3969.3975Keywords:
Soil, Cluster Analysis, Factorial Analysis, PCA, mining activity, source apportionmentAbstract
The present study was conducted to evaluate the source apportionment of soil pollution and its impact on soil quality using multivariate statistical analysis tools. Multivariable statistical techniques such as cluster analysis (CA), principal component analysis (PCA), factor analysis (FA) and correlation offers superior interpretation of complicated data sets to better understand the soil quality. Soil samples were analyzed for physicochemical parameters (pH, temperature, electrical conductivity, organic carbon, moisture content, phosphate, potassium and sulphar) and for some important heavy metals (zinc, iron, manganese and copper). Sampling stations have been classified into three groups using Cluster Analysis (CA) in a convincing way. Results revealed that among all the sampling stations, three significantly different groups: (1) stations having highest pollution sources (HW and MW), (2) Moderate polluted area (SW and IW) & (3) control site (VW) of the study stretch. The PCA generated 2 significant factors having eigenvalues >1 which explain 82.78% of the variance in the dataset. Each soil quality parameter with strong correlation coefficient value was considered to be significant (p<0.01). Therefore, the present study revealed that mining activities, dumping of municipal sewage, disposal of biomedical waste and industrial effluent including solid waste (e.g fly ash) have greater influence on the soil quality. Results also showed that the concentrations of heavy metals (Cu, Mn and Zn) in highly polluted soil are beyond the permissible limit. The pollutant levels were significantly different in the three groups of soil pollution character samples, which were confirmed by ANOVA analysis.
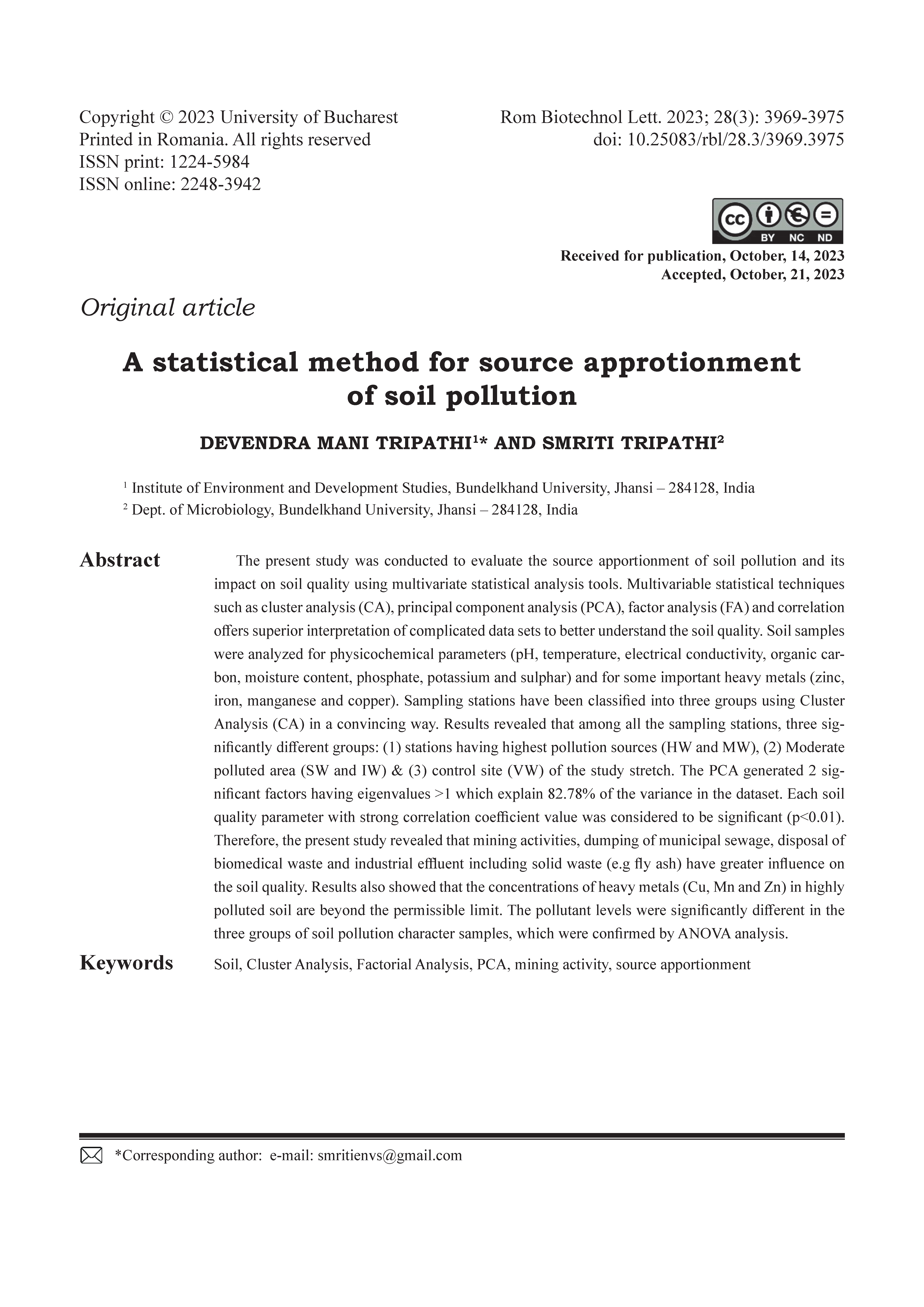